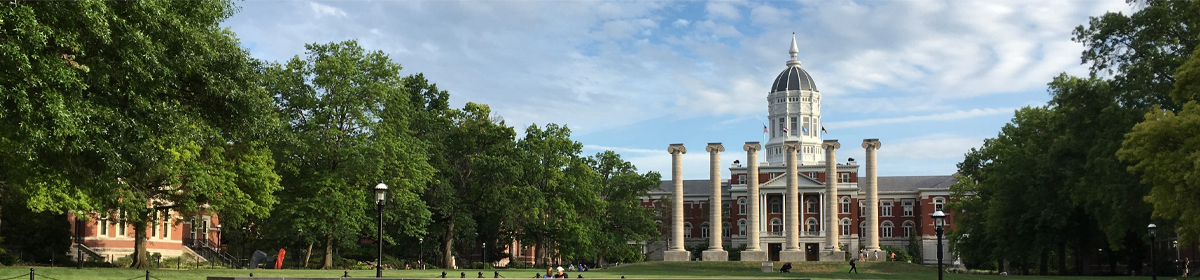
Behavioral Learning of a Fuzzy Decision-Maker
2015 MU IEEE Computational Intelligence Society Poster Contest
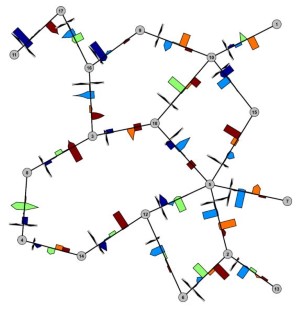
Abstract
In this project, we aim to understand the behavior of a decision-making agent. In particular, we present the agent with an environment, represented as a fuzzy weighted graph, and observe the preferred routes between any two points in the graph. We assume the agent follows the principle of bounded rationality, implemented as an alpha-level OWA operator, to determine the path with the smallest perceived cost. We design an experiment to generate many such agents and environments, and to observe the preferred route between any two points. The agent parameters are then learned from the observed data using a genetic algorithm. We present our findings, in which we show a high degree of reproducibility between the original and learned agent.
Related Work