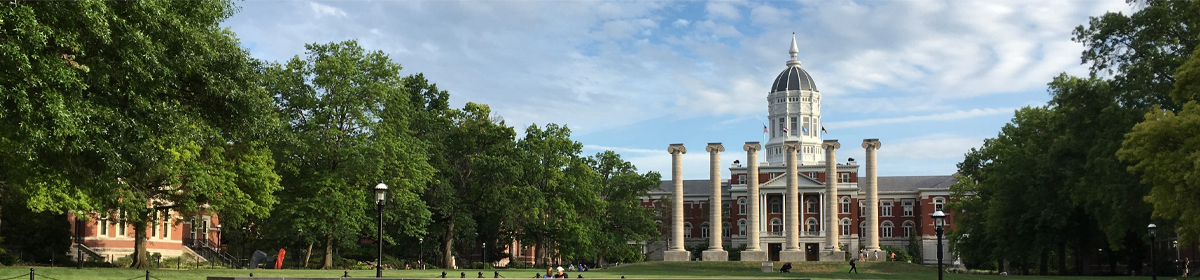
Improving Explosive Hazard Detection with Simulated and Augmented Data for an Unmanned Aerial System
Proc SPIE 11750, Detection and Sensing of Mines, Explosive Objects, and Obscured Targets XXVI, 2021
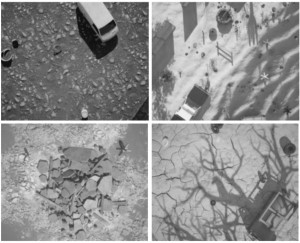
Abstract
Modern supervised machine learning for electro-optical and infrared imagery is based on data-driven learning of features and decision making. State-of-the-art algorithms are largely opaque and questions exist regarding their interpretability and generalizability. For example, what are the learned features, what contexts do they work in, and are the algorithms simply memorizing observations and exploiting unwanted correlations or has it learned an internal representation and causal associations that generalize to new environments? Under the hood, current convolutional neural networks (CNN) are sophisticated curve fitters that are sensitive to sampling (volume and variety). This is problematic as collecting data from real systems is often expensive and time consuming. Furthermore, labeling and quality checking of that data can also be prohibitive. As a result, many are looking to augmentation and simulation to efficiently generate more samples. Herein, we focus on ways to combine augmentation and simulation to improve explosive hazard detection. Specifically, we use the Unreal Engine to produce ray traced simulated data sets of environments and emplacements not captured in real data. We also present a new technique, coined altitude modulated augmentation (AMA), that inserts simulated objects into real world background imagery based on metadata to augment new training data. Thus, the goal of AMA is to increase sampling of observed environments. Preliminary results show that the combination of all techniques is best, followed by augmentation, simulation, then real world data.
Media