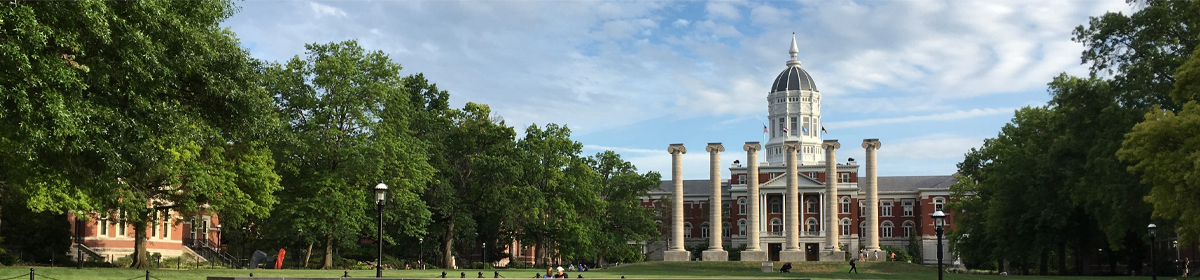
Procedurally Generated Simulated Datasets for Aerial Explosive Hazard Detection
Proc. SPIE 12116, Chemical, Biological, Radiological, Nuclear, and Explosives (CBRNE) Sensing XXIII, 2022
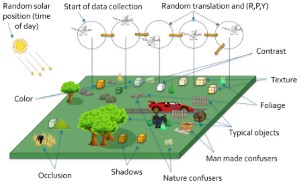
Abstract
Recent advancements in signal processing and computer vision are largely due to machine learning (ML). While exciting, the reality is that most modern ML approaches are based on supervised learning and require large and diverse collections of well annotated data. Furthermore, top performing ML models are black (opaque) versus glass (transparent) boxes. It is not clear what they are doing and when/where they work. Herein, we use modern video game engine technology to better understand and help create improved ML solutions by confronting the real world annotated data bottleneck problem. Specifically, we discuss a procedural environment and dataset collection process in the Unreal Engine (UE) for explosive hazard detection (EHD). This process is driven by the underlying variables impacting EHD: object, environment, and platform/sensor (low altitude drone herein). Furthermore, we outline a process for generating data at different levels of visual abstraction to train ML algorithms, encourage improved features, and evaluate ML model generalizability. Encouraging preliminary results and insights are provided relative to simulated aerial EHD experiments.
Media