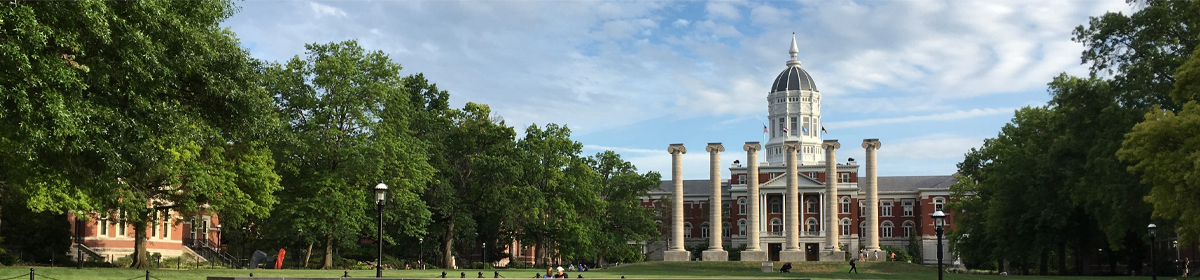
Capturing Uncertainty in Monocular Depth Estimation: Towards Fuzzy Voxel Maps
2023 IEEE International Conference on Fuzzy Systems (FUZZ-IEEE), Songdo Incheon, Korea, 2023
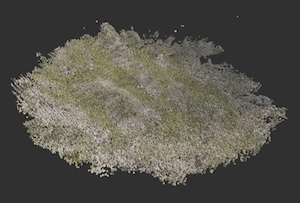
Abstract
Monocular depth estimation methods using structure from motion (SfM) have become increasingly capable of reconstructing 3D representations from a sequence of 2D images. In the context of unmanned aerial vehicles (UAVs), these techniques can be used to create an occupancy map of an environment, which is useful for planning and navigation. OctoMap and a recent improvement, UFOMap, are commonly used hierarchical representations that represent the value of a voxel cell as the probability of being occupied. Although this captures some uncertainty in the map and allows for dynamic updates, it does not fully utilize the known characteristics of the sensor and SfM algorithm, and it can lead to unnecessarily noisy results. In this paper, we propose an approach to assign a weight to each point in a point cloud update based on camera extrinsics and SfM confidence. The weighted points are then added to the voxel map in a way that more closely resembles a degree of confidence rather than a probability. In this way, we take the first steps toward designing a fuzzy voxel map that is more robust in noisy situations and captures useful uncertainty to help with UAV applications. We demonstrate our approach on simulated scenarios using Unreal Engine and AirSim.