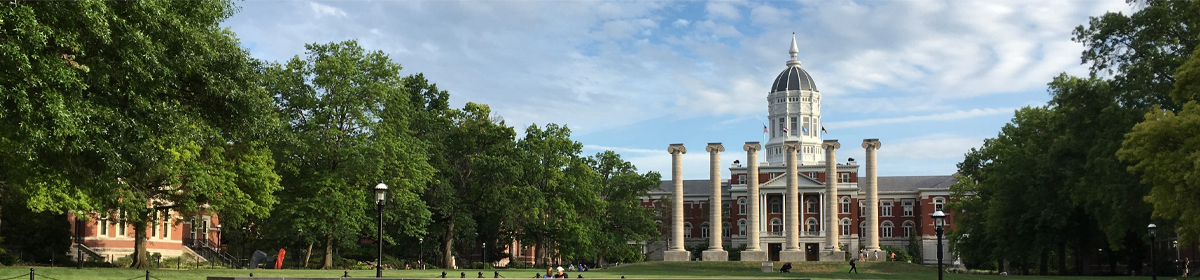
HiFiAerial: A Photorealistic Synthetic Dataset with Ground Truth for Dense Prediction in Aerial Imagery
Proc. SPIE 13459, Synthetic Data for Artificial Intelligence and Machine Learning: Tools, Techniques, and Applications III, 2025
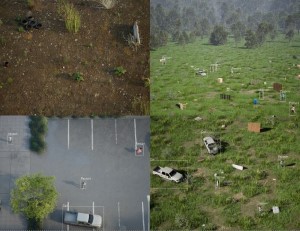
Abstract
Training Deep Neural Networks (DNNs), particularly modern architectures such as the Transformer, is an incredibly intensive task which requires large quantities of data. However, collecting precise, real-world data can be extremely challenging, if not impossible for certain tasks where dense, per-pixel labels are required. In lieu of real-world data of sufficient quality for these tasks, models trained on high-quality simulated/synthetic data have been shown to outperform models trained on a larger corpus of real-world data, e.g., Depth Anything V2. Despite this, there is a lack of high-quality (photorealistic) publicly-available datasets for aerial contexts, which is necessary for training detection, tracking, and 3D estimation models for unmanned aerial vehicles (UAVs), micro-drones, or other low-to-medium-altitude aircraft. Herein, we present HiFiAerial, an open source collection of simulated image sequences extracted from a diverse selection of photorealistic urban and rural biomes in Unreal Engine 5 at low/medium/high altitudes with both nadir and off-nadir relative viewing angles while traversing random paths. Each sequence is accompanied by a comprehensive set of dense labels (metric depth, object AABBs, etc.), camera poses (location, roll, pitch, yaw), camera intrinsics, and other metadata. While we showcase algorithm performance on this dataset, the broader goal is to empower other researchers and foster community-driven benchmarking experiments. The dataset is available at https://github.com/MizzouINDFUL/HiFiAerial.